Human-AI tools for accounting for differences across contexts
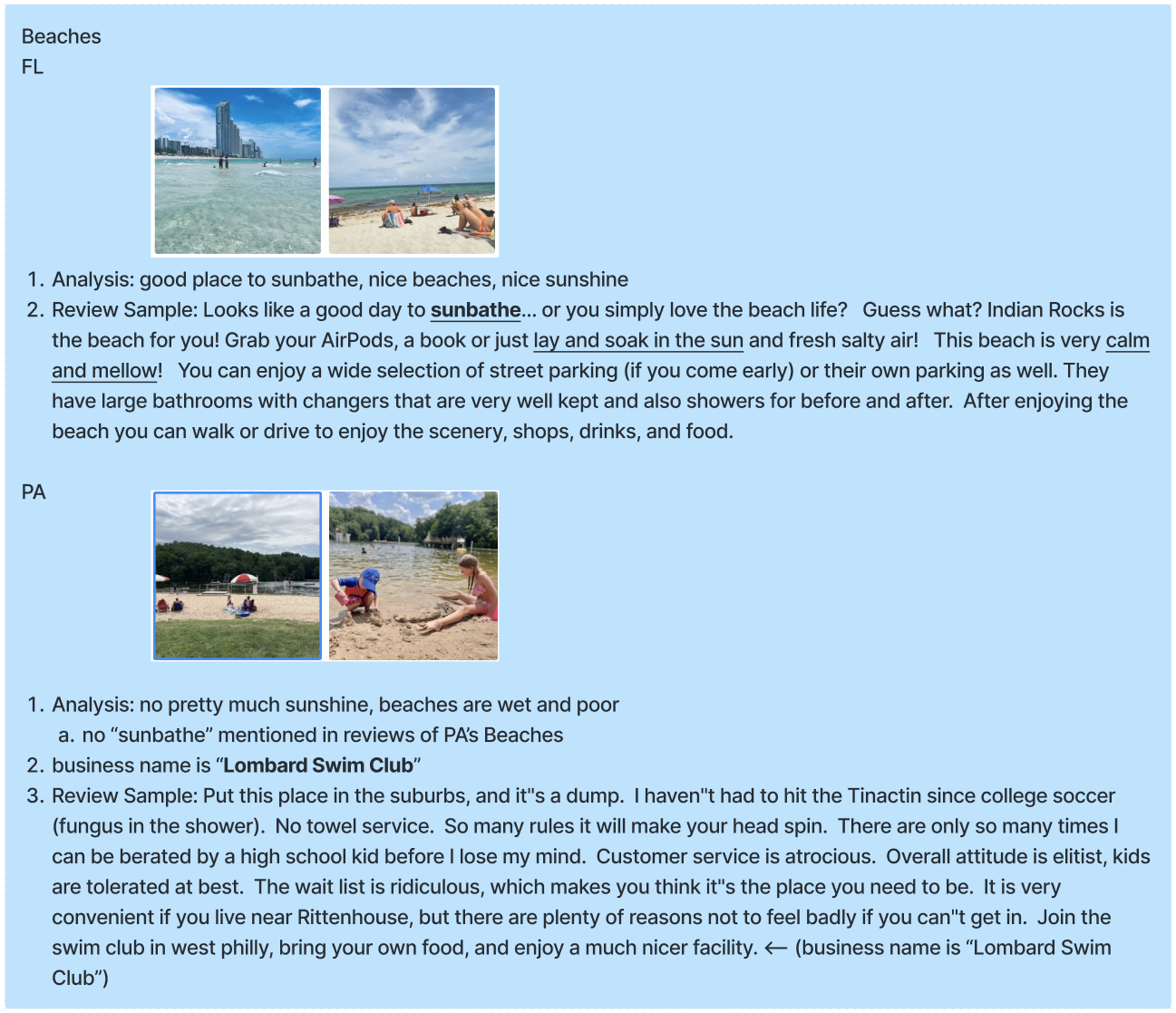
Addressing the challenge of context-aware computing’s failure to fully appreciate and adapt to the diverse geographical, social, and cultural differences across settings, we introduce a novel system that utilizes a differentiated Term Frequency-Inverse Document Frequency (TF-IDF) model along- side a user-centric interface. At the heart of our solution is the ability to dynamically recognize and adapt to the unique cultural and social nuances of various regions, thereby making AI-driven solutions more relevant, accessible, and inclusive. This paper details the design, implementation, and evaluation of our system, focusing on its capacity to assist users in generating culturally and geographically sensitive concept queries, accurately identify expected categories, and facilitate the ideation process. Through a comprehensive study involving participants from diverse backgrounds, the results demonstrate a significant improvement in user engagement, conceptual accuracy, and the generation of innovative ideas. These findings validate our system’s effectiveness in bridging the gap between human concept expression and machine-understandable context features, thereby addressing the critical need for adaptability, equity, and inclusion in AI-driven experiences across varied settings.
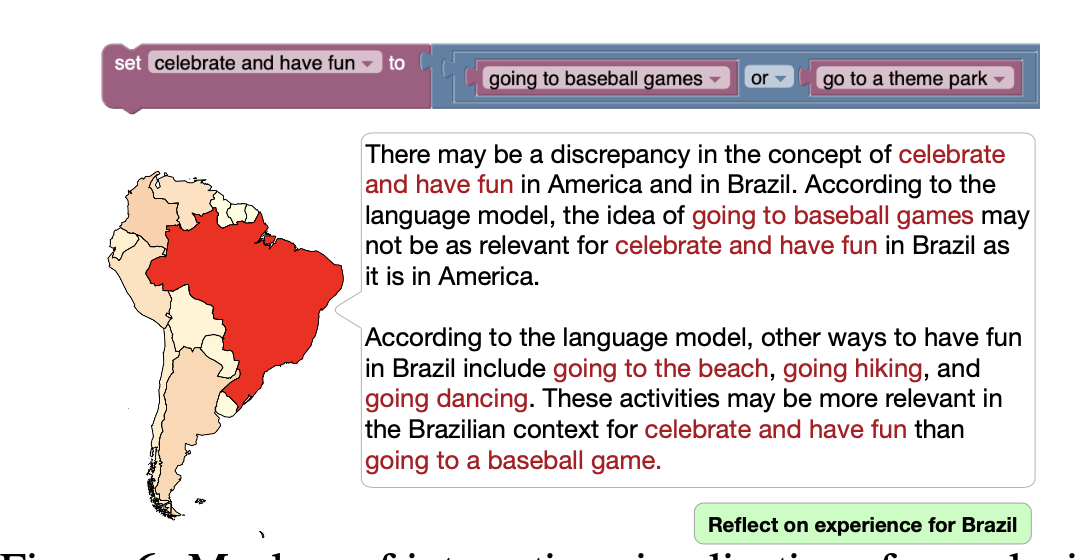
Figure 1: Example 1 and 2
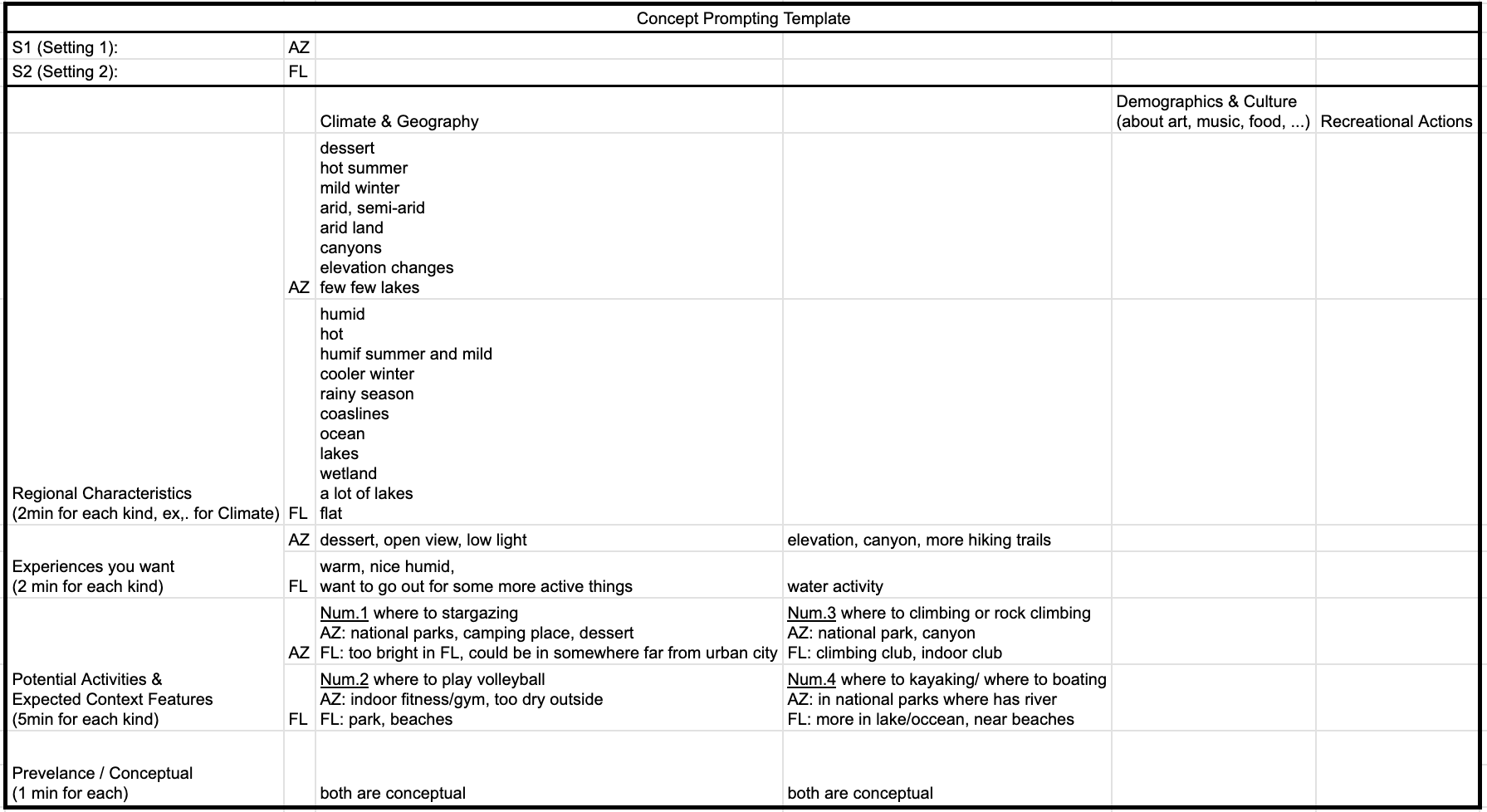
Figure 2: Concept Prompting Template and Results for "where to stargazing"
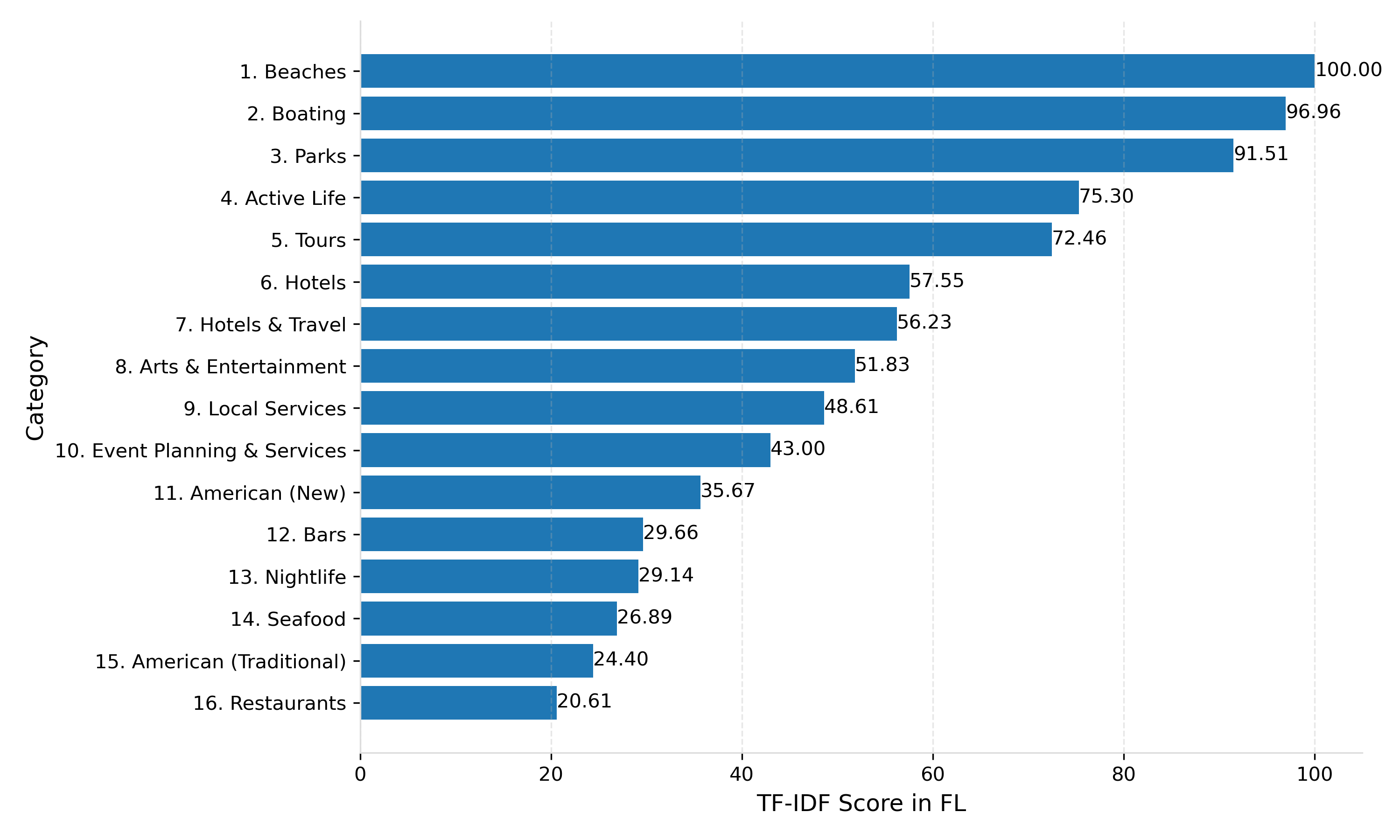
Figure 3: Figure 1: Categories in FL related to ’Where to Sunbathe’
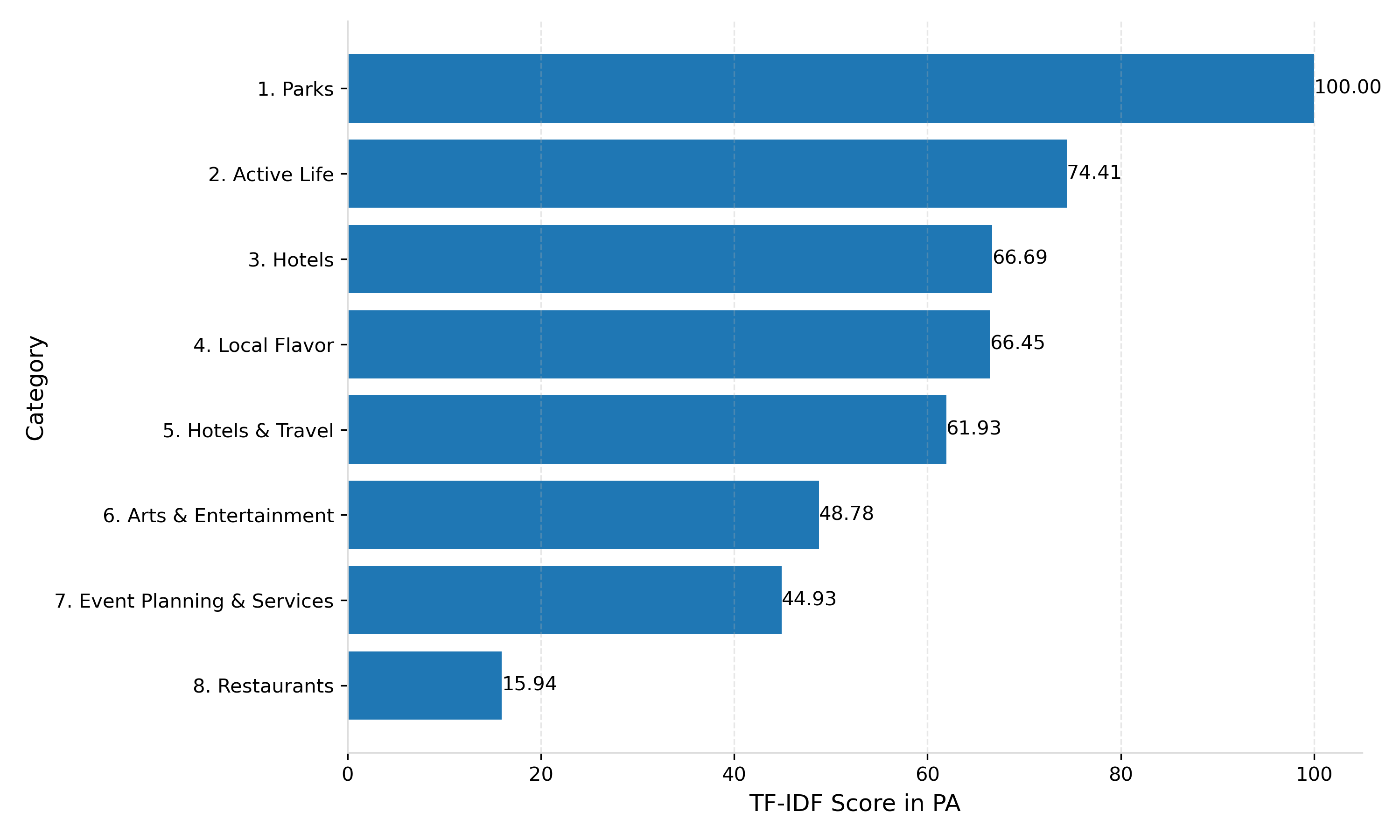
Figure 4: Figure 2: Categories in PA related to ’Where to Sunbathe’
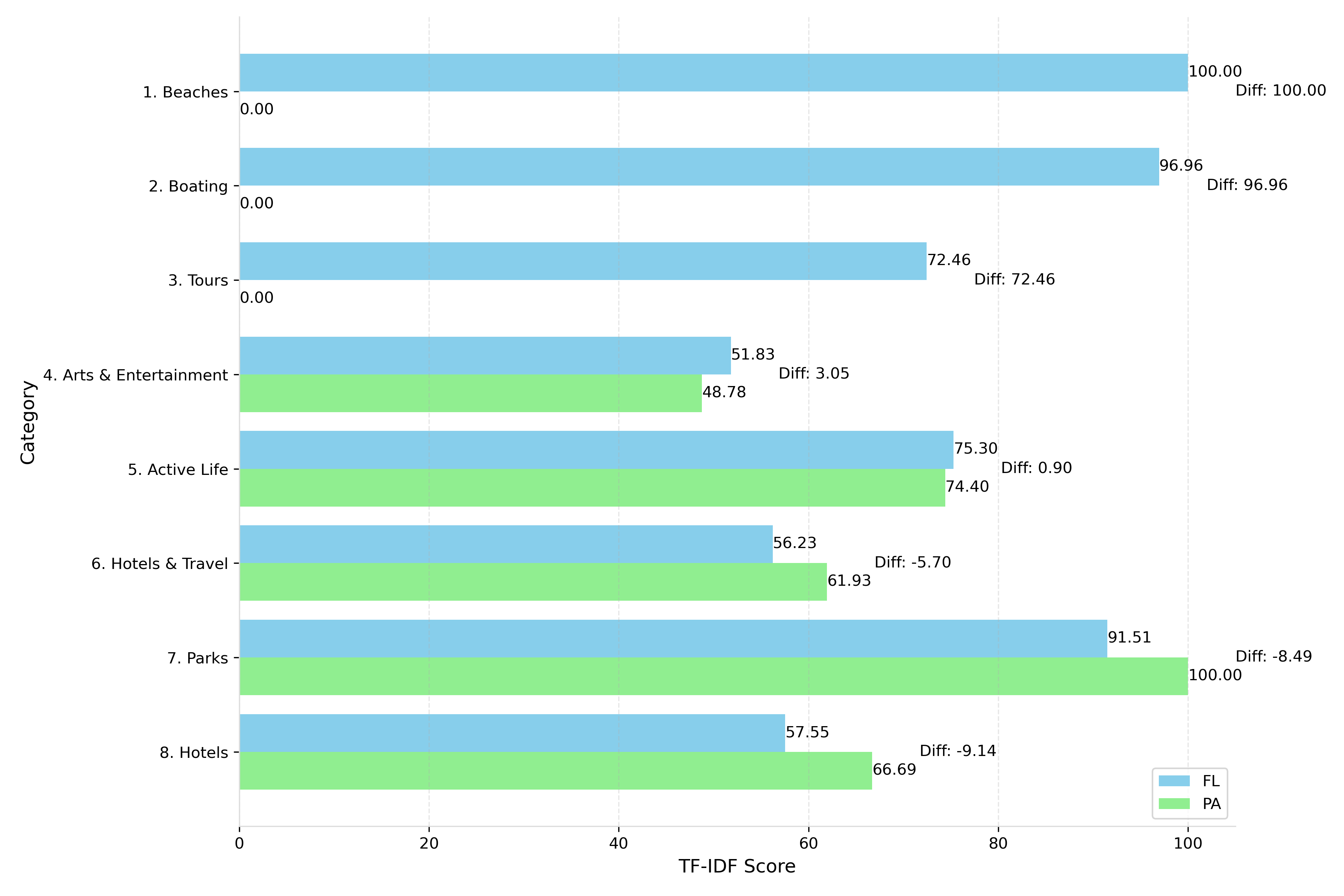
Figure 5: Figure 3: Comparative Analysis of TF-IDF Scores by Category to ’Where to Sunbathe’ in FL and PA
Publications
- A Human-Centered Classifier for Cross-Setting Differences, DTR Deliverable - Spring 2023
- Accounting for Differences: AI’s Role in Contextual and Cultural Analysis to a Concept Expression, DTR Deliverable - Fall 2023
- Adapting to Diversity: Enhancing Context-Aware AI Through Regional Sensitivity and Automated Analysis, DTR Deliverable - Winter 2024
Team
Faculty
- None
Ph.D. Students
- None
Masters and Undergraduate Students
- 🎓 Jiayi Zheng
- 🎓 Yiran Mo
- 🎓 Suhuai Chen